The man behind Nvidia’s lead in the AI race
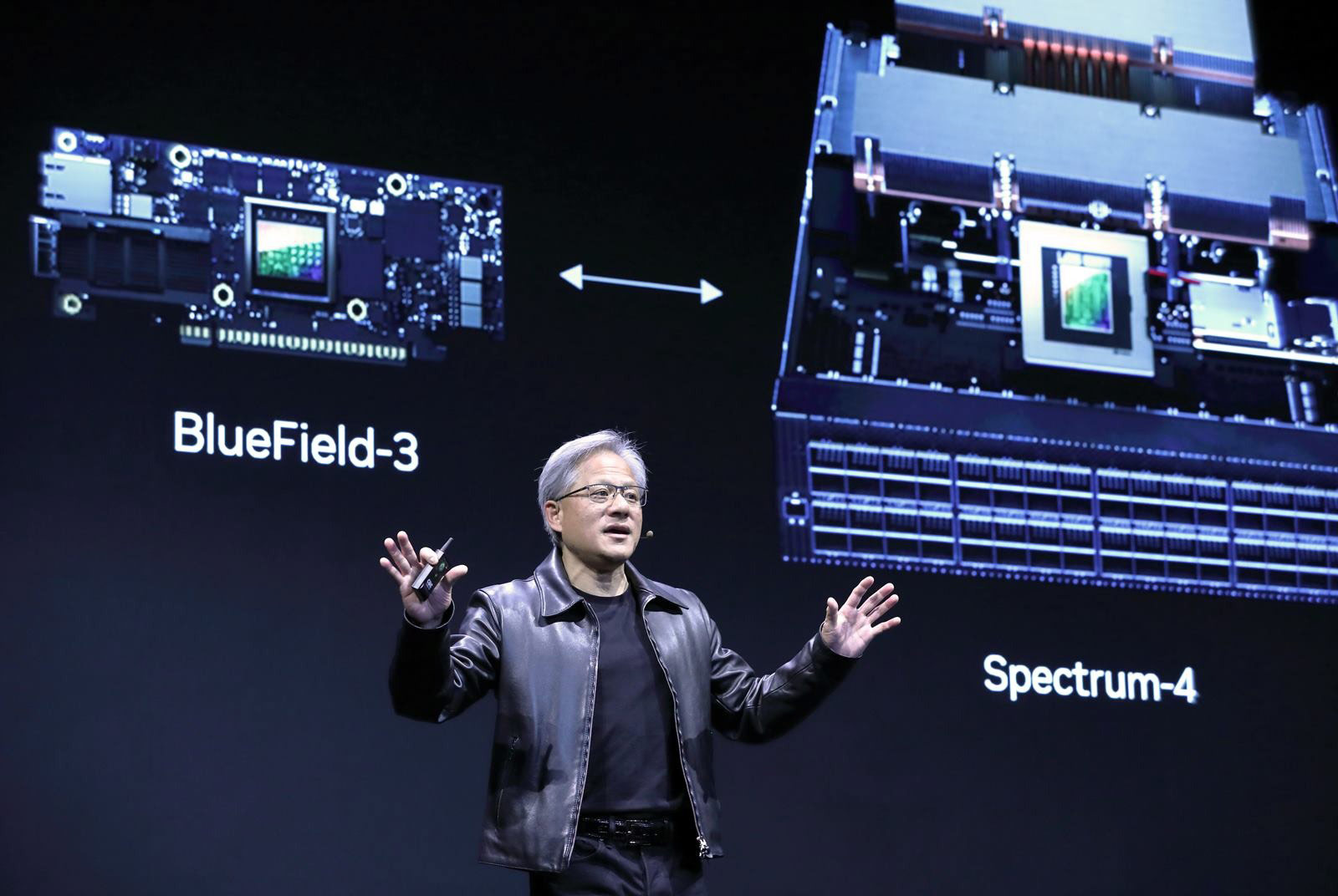
Source:Chien-Tong Wang
At Computex Taipei on 5/29, Nvidia CEO Jensen Huang's keynote speech was packed with 4,000 audience members. From a graphics card company focusing on the gaming industry to the largest graphics processor provider behind generative AI, how did Nvidia, whose stock price has soared, reach the top of AI?
Views
The man behind Nvidia’s lead in the AI race
By Elaine Huangweb only
"Don’t say again that we are expensive," declared Jensen Huang, founder and CEO of California-based chipmaker Nvidia, with great fanfare as he addressed a 4,000-strong audience at the Computex computer show in Taipei on May 29.
Presenting on stage in his trademark black leather jacket, Tainan-native Huang explained that it takes a data center with 960 CPU servers at a cost of US$10 million to train a large language model.
Switching to another slide, Huang pointed out that two servers using Nvidia GPUs can achieve the same performance at a markedly lower cost of US$400,000.
He deflected the complaints about Nvidia GPUs, which dominate the market for AI model training, being far more expensive than CPUs, saying that one Nvidia GPU performs better than 480 CPUs.
Jensen Huang demonstrates the Nvidia song generated by AI. (Source: Chien-Tong Wang)
On May 24, Nvidia adjusted its second-quarter revenue forecast upward to US$11 billion, some 50 percent ahead of the Wall Street estimate of US$7 billion. As a result, the share price soared, pushing Nvidia’s market value to nearly US$1 trillion, which makes it the sixth-largest company worldwide in terms of market value.
It also catapulted Huang, who currently holds a 3.5 percent stake in the company, into the ranks of the world’s 50 richest people. Thanks to the AI craze, Huang’s net worth has risen to US$27.6 billion, netting him rank 37 among the world’s billionaires.
Nvidia, which started out as a graphics card manufacturer, has always thrived in the gaming industry and later benefited from the bitcoin mining craze. Subsequently, the chipmaker developed its parallel computing platform and programming model CUDA (Compute Unified Device Architecture), which, as it turned out, can be used to optimize GPUs for training artificial intelligence and deep learning models.
Bryan Catanzaro, vice president of Applied Deep Learning Research at Nvidia, is the brain behind the company’s venture into AI.
Catanzaro joined Nvidia as a researcher in 2011, having just completed his Ph.D. in electrical engineering at the University of California, Berkeley. He sensed that machine learning would change the world and that accelerated computing was crucial for unleashing the full potential of machine learning.
After joining Nvidia, Catanzaro focused on parallel computing programming and modeling design and the creation of a software library for AI-driven deep learning. He established the CUDA Deep Neural Network library (cuDNN) to enable GPUs for accelerated computing, a prerequisite for deep learning.
"That work led to the creation of cuDNN, which was really NVIDIA's first software product targeted for deep learning.," recalls Catanzaro.
Li Yung-hui, director of the Artificial Intelligence Research Center of Hon Hai Research Institute, points out that deep learning software has many layers. While GPUs constitute the lowest hardware layer, Nvidia’s GPUs have an intermediate layer, which is the "CUDA" software platform developed by Nvidia. Once the cuDNN library has been created, Google’s machine learning platform TensorFlow, Facebook’s machine learning platform PyTorch, and other deep learning software can utilize cuDNN functions to perform accelerated computing on GPUs.
Back then, AlphaGo, the Go-playing computer, had not made its impressive debut yet, so deep learning was far from being a hot topic. At Nvidia, only a handful of computer scientists were researching artificial intelligence, and Catanzaro was the only one working on cuDNN.
Bryan Catanzaro is vice president of Applied Deep Learning Research at NVIDIA (Source: Nvidia)
Before 2011, Huang never even mentioned AI
When Catanzaro created cuDNN in 2011, Huang’s focus was not on AI at all.
In an interview with Forbes in 2016, Huang said he knew GPUs had a lot more potential than powering video games, but he did not anticipate the shift to deep learning.
A look at Huang’s keynote speeches at Nvidia’s annual GTC developer conference shows that he never mentioned AI prior to 2014.
Geoffrey Hinton, the godfather of AI, and two of his students, Alex Krzyzewski and Ilya Sutskever (founder of Open AI), won the ImageNet image recognition challenge in 2012 with the convolutional neural network AlexNet, which delivered dramatically improved performance in the field of computer vision. Their paper on AlexNet triggered massive interest in neural networks and deep learning.
Hinton said at the time that AlexNet would probably not have happened without Nvidia, notably its GPUs with more than 1000 cores computing in parallel.
At the time, Catanzaro was collaborating with AI researcher Andrew Ng on a project at Stanford University. They wanted to replace 1000 Google Brain servers with 3 Nvidia CUDA plus GPUs. In 2013, they published their paper.
At the same time, they discussed the project with New York University AI Labs’ computer scientists Yann LeCun (now Facebook’s director of AI Research) and Rob Fergus (now a research scientist at DeepMind).
Catanzaro recalls that back then Fergus told him about a group of machine learning researchers who were madly writing software for GPU cores. “You should really
look into that,” was Fergus’ advice.
Catanzaro took the advice seriously and continued to optimize the cuDNN library. He also noticed that Nvidia customers started to buy large amounts of GPUs for deep learning purposes.
And Huang began to discuss the operation of deep learning with Catanzaro.
Reportedly, Huang almost immediately became aware that this would change a lot because in the past the most difficult part of software generation was automation. Catanzaro admits that AlexNet was an important driving factor in that regard.
In his keynote speech at the GTC in 2014, Huang emphasized that "one of the most exciting applications in high-performance computing today is a field that started practically 50 years ago: machine learning." He went on to say that "one of the areas that has seen recent breakthroughs, enormous breakthroughs, magical breakthroughs, simply amazing results, is an area called deep neural nets."
Jensen Huang, who had not talked about AI before 2014, accelerated the research and development of overtaking, and came to an AI sweet spot at this time. (Source: Chien-Tong Wang)
Chipmaker backed up by a large software team
Nvidia is strengthening the creation of deep learning libraries to enhance communication among the more than 1000 cores on its GPUs for even better performance. Efforts particularly focus on CUDA toolkits such as cuDNN.
Due to its software-focused strategy, Nvidia employs a large number of software engineers.
An executive with a system maker who is familiar with Nvidia’s organizational setup told CommonWealth Magazine that one team is tasked with chip design while another team works on software so that software and hardware work well with the architecture.
Catanzaro confirms that the microchips themselves are not the secret behind the strong computing power of Nvidia GPUs.
“We believe that a chip by itself is just sand unless it has the software written along with it and co-optimized with that chip. And not just the software, but also the systems. So accelerated computing for NVIDIA means that we have more people working on software than on chips.
“When we talk about accelerated computing, the secret is that it's not a chip,” explains Catanzaro, adding that the concept is still little understood. “It's the kind of secret that you can tell people over and over again and they still don't understand.”
(Source: Chien-Tong Wang)
A high-ranking MediaTek manager notes that "Nvidia has a very complete ecosystem from hardware to software and system." He points out that Nvidia’s marketing channel strategy is to enable customers to choose the most suitable solution and resources within the Nvidia platform and ecosystem.
After 2018, Nvidia GPU products penetrated the field of AI. Aside from training models, they are also used for another AI core area – deduction. With the advent of generative AI, the training of large language models depends even more on computing power. Mass production of the GPU supercomputer H100 will start this year, putting Nvidia further ahead of its competitors Intel and AMD.
Anyone in the generative AI arms race, be it Microsoft, Google, or Meta, needs Nvidia’s GPU supercomputers. And the reach of generative AI is going to drive Nvidia’s next growth wave.
Have you read?
- Nvidia Founder Jensen Huang's Path to Success
- Can Taiwan Find an AI Niche?
- AI medical records assistant Copilot
Translated by Susanne Ganz
Uploaded by Ian Huang